Coupled Taguchi-Pareto-Box Behnken Design-Grey Wolf Optimization Methods for Optimization Decisions when Boring IS 2062 E250 Steel Plates on CNC Machine
doi: 10.14456/mijet.2024.4
Keywords:
Grey wolf optimisation, CNC machine, Taguchi-Pareto, fitness values, parametersAbstract
Optimizing process parameters in boring operation is extremely important as aids to maintain high resource conservation and efficiency and the free flow of boring data while optimally using boring resources. With optimal parameters, real-time information is offered to process engineers for the practical control the boring operation thus reducing the cost of boring operations. This article presents an investigation on the use of optimization and prioritization in the boring process of IS 2062 E250 steel plates using coupled Taguchi-Pareto-Box Behnken Design-grey wolf optimization approach. The experimental data, drawn from the literature, was initially provided by Patel and Deshpande on CNC TC machine. The objective function, constraints, population size, number of iterations, and fitness function were determined. Then the solution for the grey wolf optimization is generated using the python programming language. Three representative parameters of speed, feed and depth of cut were foundations of the Taguchi’s experimental design used for solving the problem. The optimal parameters were determined from the experiments. For the first time, the coupled Taguchi-Pareto-Box Behnken Design-grey wolf optimization method to make optimization decisions for the boring process. Using 50 iterations and 200 wolfs, the best fitness value of wolves at the end of the 50th iteration is 872728.53 when the objective function is generated from optimized Box Behnken Design parameters. It also has an optimal solution of speed, feed, depth of cut and nose radius as 800rpm, 0.06,1 and 0, respectively. However, on the application of the regression equation from the Box Behnken Design to form an objective function, using the same 50 iteration and 200 wolves the best fitness value of wolves at the end of 50th iteration is -51.49 while the optimal parameters are 1189.58, 0.089, 1.22, 0.55 for the speed, feed, depth of cut and nose radius, respectively. Hence, the outcome of this study may be a route to reducing time and money associated with unnecessary usage of non-optimal boring data during operations planning decisions.
References
D.D. Trung, “The combination of taguchi entropy-WASPAS-PIV methods for multi-criteria decision making when external cylindrical grinding of 65G steel,” Journal of Machine Engineering, vol. 21, no. 4, pp. 90-105, 2021.
S. Sivarajan, M. Elango, M. Sasikumar, A.S.A. Doss, “Prediction of surface roughness in hard machining of EN31 steel with TiAlN coated cutting tool using fuzzy logic,” Materials Today: Proceedings, vol. 65, no. 1, pp. 35-41, 2022. https://doi.org/10.1016/j.matpr.2022.04.161
P. Kittali, V. Kalwa, D. Athith, K.P. Prashanth, B.K. Venkatesh, “Optimization of machining parameters in turning operation to minimize the surface roughness using Taguchi technique for EN1A alloy steel,” Materials Today: Proceedings, vol. 54, no. 2, pp. 463-467, 2022. https://doi.org/10.1016/j.matpr.2021.10.323
M.P. Motta, C. Pelaingre, A. Delamézière, L.B. Ayed, C. Barlier, “Machine learning models for surface roughness monitoring in machining operations,” Procedia CIRP, vol. 108, pp. 710-715, 2022. https://doi.org/10.1016/j.procir.2022.03.110
C.C. Sastry, P. Hariharan, M.P. Kumar, “Experimental investigation of dry, wet and cryogenic boring of AA 7075 alloy,” Materials and Manufacturing Processes, vol. 34, no. 7, pp. 814–831, 2019. https://doi.org/10.1080/10426914.2019.1605174
C.C. Sastry, K. Gokulakrishnan, P. Hariharan, M.P. Kumar and S.R. Boopathy, “Investigation of boring on gunmetal in dry, wet and cryogenic conditions,” Journal of the Brazilian Society of Mechanical Sciences and Engineering, vol. 42, no. 16, 2020. https://doi.org/10.1007/s40430-019-2091-2
I.D. Marinescu, M.P. Hitchiner, E. Uhlmann, W.B. Rowe, I. Inasaki, “Handbook of Machining with Grinding Wheels”, CRC Press, USA, 2006.
K. Singh and I. Sultan, “Parameters optimization for sustainable machining by using taguchi method,” Materials Today: Proceedings, vol. 18, no. 7, pp. 4217-4226, 2019.
https://doi.org/10.1016/j.matpr.2019.07.380
S. Venkatesh, S. Prakash, R.B. Durairaj, M.R. Vimal, L. Gaind, P. Chaturvedi, “Machinability of SUPERNI-800 during PMEDM using the taguchi method,” Materials Today: Proceedings, vol. 44, no. 5, pp. 3851-3855, 2021. https://doi.org/10.1016/j.matpr.2020.12.823
K.M. Senthilkumar, R. Thirumalai, T.A. Selvam, A. Nataraj and T. Ganesane, “Multi objective optimization in machining of Inconel 718 using taguchi method,” Materials Today: Proceedings, vol. 37, no. 2, pp. 3466-3470, 2021. https://doi.org/10.1016/j.matpr.2020.09.333
B.J. Kadam, K.A. Mahajan, “Optimization of cutting temperature in machining of titanium alloy using response surface method, genetic algorithm and taguchi method,” Materials Today: Proceedings, vol. 47, no. 17, pp. 6285-6290, 2021. https://doi.org/10.1016/j.matpr.2021.05.252
P. Quitiaquez, J. Cocha, W. Quitiaquez, X. Vaca, “Investigation of geometric parameters with HSS tools in machining polyamide 6 using taguchi method,” Materials Today: Proceedings, vol. 49, no. 1, pp. 181-187, 2022. https://doi.org/10.1016/j.matpr.2021.08.002
R. Vasanth, K. Mohan, S. Rengarajan, R. Jayaprakash and R.A. Kumar, “Characterization and corrosion effects of friction surfaced IS-2062 E250 CU with AA6063,” Materials Research Express, vol. 6, no. 12, pp. 1-9, 2019.
N. T. Patel, N. A. Deshpande, “Application of taguchi approach for optimization roughness for boring operation of E 250 B0 for Standard IS: 2062 on CNC TC,” International Journal of Engineering Development and Research, vol. 2, no. 2, pp. 2528-2537, 2014.
G. Kant, K.S. Sangwan, “Prediction and optimization of machining parameters for minimizing power consumption and surface roughness in machining,” Journal of Cleaner Production, vol. 83, pp. 151-164, 2014. https://doi.org/10.1016/j.jclepro.2014.07.073
M. Sekulic, V. Pejic, M. Brezocnik, M. Gostimirović, M. Hadzistevic, “Prediction of surface roughness in the ball-end milling process using response surface methodology, genetic algorithms, and grey wolf optimizer algorithm,” Advances in Production Engineering & Management, vol. 13, no. 1, pp. 18-30, 2018. https://doi.org/10.14743/apem2018.1.270
C.G. Burande, O.K. Kulkarni, S. Jawade, G.M. Kakandikar, “Process parameters optimization by bat inspired algorithm of CNC turning on EN8 steel for prediction of surface roughness,” Journal of Mechatronics and Artificial Intelligence in Engineering, vol. 2, no. 2, pp. 73-85, 2021. https://doi.org/10.21595/jmai.2021.22148
M. Hanief, M.F. Wani, M.S. Charoo, “Modeling and prediction of cutting forces during the turning of red brass (C23000) using ANN and regression analysis,” Engineering Science and Technology, an International Journal, vol. 20, no. 3, pp. 1220-1226, 2017. https://doi.org/10.1016/j.jestch.2016.10.019
P.K. Kharwar, R.K. Verma, “Nature instigated grey wolf algorithm for parametric optimization during machining (milling) of polymer nanocomposites,” Journal of Thermoplastic Composite Materials, vol. 36, no. 1, pp. 118-140, 2021. https://doi.org/10.1177/0892705721993202
S.R. Mangaraj, D.K. Bagal, N. Parhia, S.N. Panda, A. Barua, S. Jeet, “Experimental study of a portable plasma arc cutting system using hybrid RSM-nature inspired optimization technique,” Materials Today: Proceedings, vol. 50, no. 5, pp. 867-878, 2022. https://doi.org/10.1016/j.matpr.2021.06.138
O. Kulkarni, S. Jawade, G. Kakandikar, “Parameter optimization of AISI 316 austenitic stainless steel for surface roughness by grasshopper optimization algorithm,” Journal of Mechanical Engineering, Automation and Control Systems, vol. 2, no. 2, pp. 1-11, 2021. https://doi.org/10.21595/jmeacs.2021.22149
J.X. Mary, M.A. Balaji, A.D. Selvakumar, D. Devaraj, “Adaptive control of tool wear by greywolf optimization and neural controller in drilling,” International Journal of Robotics and Automation, vol. 36, no. 1, pp. 1-6, 2020. https://doi.org/10.2316/J.2021.206-0436
S. Chakraborty and A. Mitra, “Parametric optimization of abrasive water-jet machining processes using grey wolf optimizer”, Materials and Manufacturing Processes, vol. 33, no. 13, pp. 1471-1482, 2018. https://doi.org/10.1080/10426914.2018.1453158
L. Imani, A.R. Henzaki, R. Hamzeloo, B. Davoodi, “Modeling and optimizing of cutting force and surface roughness in milling process of Inconel 738 using hybrid ANN and GA,” Proceedings of the Institution of Mechanical Engineers, Part B: Journal of Engineering Manufacture, vol. 234, no. 5, pp. 920–932, 2020. https://doi.org/10.1177/0954405419889204
O. Kulkarni, S. Kulkarni, “Process parameter optimization in WEDM by grey wolf optimizer,” Materials Today: Proceedings, vol. 5, no. 2, 2018, pp. 4402-4412, 2018.
https://doi.org/10.1016/j.matpr.2017.12.008
A. Ramalingam, S. Muthuvel, R. Mohan, “Application of grey taguchi-based response surface methodology (GT-RSM) for optimizing the plasma arc cutting parameters of 304L stainless steel,” International Journal of Advanced Manufacturing Technology, vol. 78, nos. 5-8, pp. 1161–1170, 2015. https://doi.org/10.1007/s00170-014-6744-0
S. Khalilpourazari, S. Khalilpourazary, “Optimization of production time in the multi-pass milling process via a robust grey wolf optimizer,” Neural Computing and Applications, vol. 29, pp. 1321–1336, 2018. https://doi.org/10.1007/s00521-016-2644-6
S. Khalilpourazari, S. Khalilpourazary, “Optimization of time, cost and surface roughness in grinding process using a robust multi-objective dragonfly algorithm,” Neural Computing and Applications, vol. 32, pp. 3987–3998, 2020. https://doi.org/10.1007/s00521-018-3872-8
Ö. Seçgin, “Multi-objective optimization of MS58 brass machining operation by multi-axis CNC lathe,” Arabian Journal for Science and Engineering, vol. 46, pp. 2133–2145, 2021. https://doi.org/10.1007/s13369-020-04984-8
D.K. Pradhan, B. Sahu, D.K. Bagal, A. Barua, S. Jeet, S. Pradhan, “Application of progressive hybrid RSM-WASPAS-grey wolf method for parametric optimization of dissimilar metal welded joints in FSSW process,” Materials Today: Proceedings, vol. 50, no. 5, pp. 766-772, 2022. https://doi.org/10.1016/j.matpr.2021.05.471
N.A. Fountas, A. Koutsomichalis, J.D. Kechagias, N.M. Vaxevanidis, “Multi-response optimization of CuZn39Pb3 brass alloy turning by implementing grey wolf algorithm,” Frattura ed Integrità Strutturale, vol. 50, no. 1, pp. 584-594, 2019. https://doi.org/10.3221/IGF-ESIS.50.49
Y.U. Abdullahi and S.A. Oke, “Optimizing the boring parameters on CNC machine using IS 2062 E250 steel plates: Taguchi-Pareto-Box Behnken design and Taguchi-ABC-Box Behnken design perspectives, Engineering Access, vol. 8, no. 2, pp. 219-241, 2022. https://doi.org/10.14456/mijet.2022.28
E. Fasina, B.A. Sawyerr, Y.U. Abdullahi and S.A. Oke, “A comparison of two hybrid optimization techniques: the Taguchi‑BBD‑firefly and the Taguchi‑regression‑firefly methods on the IS 2062‑E250 steel plates boring problem,” Journal of Engineering and Applied Science, vol. 70, no. 47, 2023. https://doi.org/10.1186/s44147-023-00215-7
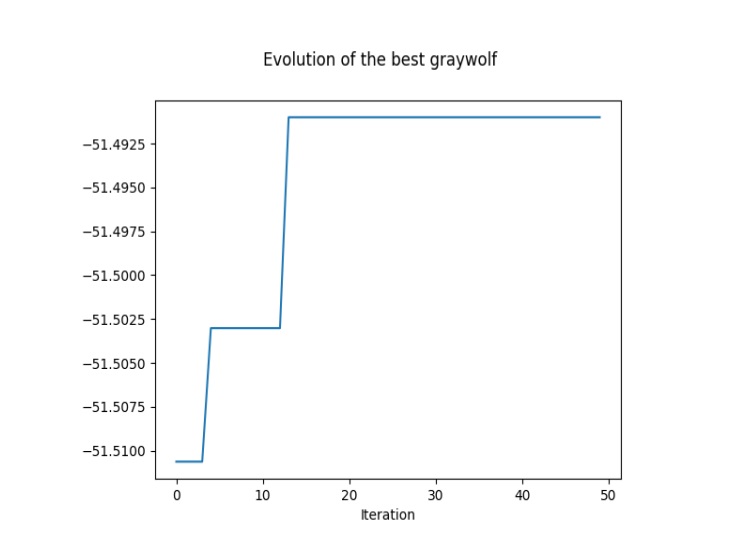
Downloads
Published
How to Cite
Issue
Section
License

This work is licensed under a Creative Commons Attribution-NonCommercial-NoDerivatives 4.0 International License.