การประยุกต์ใช้อากาศยานไร้คนขับติดตามการเจริญเติบโตของหญ้าเนเปียร์
Application of unmanned aerial vehicles to track the growth of Napier grass
DOI:
https://doi.org/10.55674/snrujiti.v2i1.247808Keywords:
UAV, height estimation, Digital Elevation Model, Digital Terrain Model, Napier grassAbstract
การศึกษานี้มีวัตถุประสงค์เพื่อติดตามการเจริญเติบโตของหญ้าเนเปียร์พันธุ์ปากช่อง 1 ช่วงตอแรก โดยใช้ภาพถ่ายทางอากาศจากอากาศยานไร้คนขับในแปลงทดลอง พื้นที่ศึกษาตั้งอยู่ที่ ตำบลหนองอ้อ อำเภอ หนองวัวซอ จังหวัดอุดรธานี โดยถ่ายภาพทางอากาศที่ความสูงในการบิน 30 60 และ 90 เมตร ประมวลผลข้อมูลของภาพผ่าน WebOEM ทำการสุ่มตัวอย่างเพื่อรวบรวมข้อมูลจากแปลงทดลอง ค่าการประมาณความสูงของหญ้าเนเปียร์ที่ได้จากภาพถ่ายทางอากาศจากอากาศยานไร้คนขับ พบว่าความสูงในการบิน ที่เหมาะสมที่สุด คือ 90 เมตร มีค่า R² คือ 0.77 และมีค่า RMSE เท่ากับ 0.40 และประมาณความสูงของหญ้าเนเปียร์และค่าการสะท้อนแสงด้วยแบบจำลองเชิงเส้นทั่วไป พบว่าความสูงในการบินที่เหมาะสมที่สุด คือ 60 เมตร ค่าที่เหมาะสมที่สุดมีค่า R² คือ 0.77 และมีค่า RMSE เท่ากับ 0.18 จากผลการศึกษาพบว่าอากาศยานไร้คนขับสามารถใช้ติดตามการเจริญเติบโตของหญ้าเนเปียร์พันธุ์ปากช่อง 1 ที่ความสูงของการบินที่เหมาะสม คือ 90 เมตรให้ค่า R² เท่ากับการวิเคราะห์ด้วยค่าการสะท้อนแสงซึ่งเป็นวิธีที่ยุ่งยากกว่า สามารถใช้เป็นแนวทางในการประมาณความสูงของหญ้าเนเปียร์พันธุ์ในพื้นที่ขนาดใหญ่ได้
References
สายัณห์ ทัดศรี, พืชอาหารสัตว์เขตร้อน. 2547, กรุงเทพฯ: สำนักพิมพ์มหาวิทยาลัยเกษตรศาสตร์.
Rengsirikul, K., et al., Biomass yield, chemical composition and potential ethanol yields of 8 cultivars of napiergrass (Pennisetum purpureum Schumach.) harvested 3-monthly in central Thailand. 2013.
กรมพลังงาน. หญ้าเนเปียร์พืชพลังงานสีเขียว 2556; Available from: Retrieved from https://webkc.dede.go.th/testmax/node/152.
สายัณห์ ทัดศรี, หญ้าอาหารสัตว์และหญ้าพื้นเมืองในประเทศไทย. 2548, กรุงเทพฯ: สำนักพิมพ์มหาวิทยาลัยเกษตรศาสตร์.
Chu, T., et al., Assessing lodging severity over an experimental maize (Zea mays L.) field using UAS images. Remote Sensing, 2017. 9(9): p. 923.
Luna, I. and A. Lobo, Mapping crop planting quality in sugarcane from UAV imagery: A pilot study in Nicaragua. Remote Sensing, 2016. 8(6): p. 500.
Na, S.-I., C.-W. Park, and K.-D. Lee, Application of highland kimchi cabbage status map for growth monitoring based on unmanned aerial vehicle. Korean Journal of Soil Science and Fertilizer, 2016. 49(5): p. 469-479.
Garcia-Ruiz, F., et al., Comparison of two aerial imaging platforms for identification of Huanglongbing-infected citrus trees. Computers and Electronics in Agriculture, 2013. 91: p. 106-115.
Moeckel, T., et al., Estimation of vegetable crop parameter by multi-temporal UAV-borne images. Remote Sensing, 2018. 10(5): p. 805.
Holman, F.H., et al., High throughput field phenotyping of wheat plant height and growth rate in field plot trials using UAV based remote sensing. Remote Sensing, 2016. 8(12): p. 1031.
Anthony, D., et al. On crop height estimation with UAVs. in 2014 IEEE/RSJ International Conference on Intelligent Robots and Systems. 2014. IEEE.
อัครพล ไชยนา and ศิวา แก้วปลั่ง, การตรวจจับโรคใบด่างในไร่มันสำปะหลังโดยใช้การสำรวจระยะไกลด้วยอากาศยานไร้คนขับ. แก่นเกษตร 2563. 48(ฉบับพิเศษ 1): p. 291-298
Kerkech, M., A. Hafiane, and R. Canals, Deep leaning approach with colorimetric spaces and vegetation indices for vine diseases detection in UAV images. Computers and electronics in agriculture, 2018. 155: p. 237-243.
Maimaitijiang, M., et al., Soybean yield prediction from UAV using multimodal data fusion and deep learning. Remote sensing of environment, 2020. 237: p. 111599.
Yang, Q., et al., Deep convolutional neural networks for rice grain yield estimation at the ripening stage using UAV-based remotely sensed images. Field Crops Research, 2019. 235: p. 142-153.
ศิวา แก้วปลั่ง, การประเมินการใช้ภาพถ่ายทางอากาศจากอากาศยานไร้คนขับสำหรับการประมาณค่าชีมวลเหนือพื้นดินของต้นหม่อน. แก่นเกษตร, 2561. 46(ฉบับพิเศษ 1): p. 381-387.
de Souza, C.H.W., et al., Mapping skips in sugarcane fields using object-based analysis of unmanned aerial vehicle (UAV) images. Computers and Electronics in Agriculture, 2017. 143: p. 49-56.
Yano, I.H., et al., Identification of weeds in sugarcane fields through images taken by UAV and Random Forest classifier. IFAC-PapersOnLine, 2016. 49(16): p. 415-420.
Geipel, J., J. Link, and W. Claupein, Combined spectral and spatial modeling of corn yield based on aerial images and crop surface models acquired with an unmanned aircraft system. Remote sensing, 2014. 6(11): p. 10335-10355.
Bendig, J., et al., Estimating biomass of barley using crop surface models (CSMs) derived from UAV-based RGB imaging. Remote sensing, 2014. 6(11): p. 10395-10412.
Shendryk, Y., et al., Fine-scale prediction of biomass and leaf nitrogen content in sugarcane using UAV LiDAR and multispectral imaging. International Journal of Applied Earth Observation and Geoinformation, 2020. 92: p. 102177.
Sofonia, J., et al., Monitoring sugarcane growth response to varying nitrogen application rates: A comparison of UAV SLAM LiDAR and photogrammetry. International Journal of Applied Earth Observation and Geoinformation, 2019. 82: p. 101878.
Wardana, K.P.W. and S. Subiyanto, ANALISIS TINGGI TANAMAN PADI MENGGUNAKAN MODEL 3D HASIL PEMOTRETAN UAV DENGAN PENGUKURAN LAPANGAN. Jurnal Geodesi Undip, 2019. 8(1): p. 378-387.
Guo, Y., et al., Scaling effects on chlorophyll content estimations with RGB camera mounted on a UAV platform using machine-learning methods. Sensors, 2020. 20(18): p. 5130.
Han, L., et al., Modeling maize above-ground biomass based on machine learning approaches using UAV remote-sensing data. Plant methods, 2019. 15(1): p. 1-19.
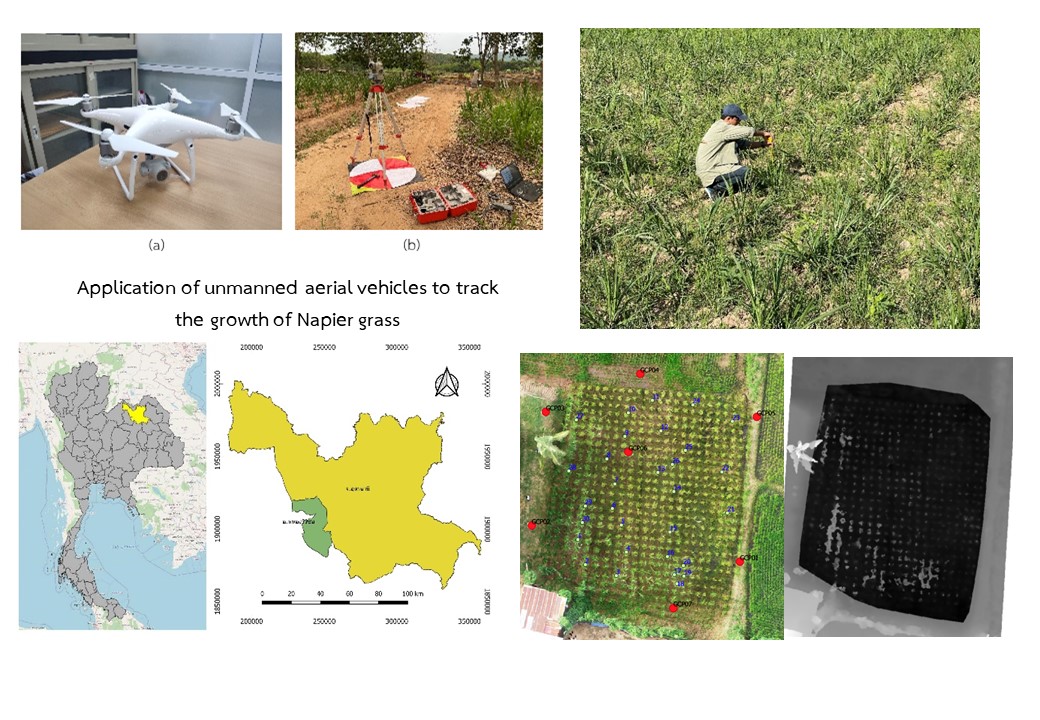
Downloads
Published
How to Cite
Issue
Section
License
Copyright (c) 2022 Journal of Industrial Technology and Innovation

This work is licensed under a Creative Commons Attribution-NonCommercial-NoDerivatives 4.0 International License.