A Multi-Criteria Approach to Maintenance Performance Assessment in a Manufacturing System
doi: 10.14456/mijet.2022.27
Keywords:
Taguchi, Grey-relational analysis, maintenance performance, fuzzy logic, artificial neural network, harmony search algorithmAbstract
Nowadays, intensive maintenance research has established procedures for evaluating the performance of industries for control and organisational planning purposes (decision making). However, decisions must be carried out in an accurate, fast and cost-effective manner in compliance with the dynamics of today's industrial activities. This brings the need for intelligent systems for control. Maintenance performance has huge metrics, and the question of how to track these measures in the circumstance of interwoven indices is an urgent problem for all maintenance systems. A novel intelligent approach was developed using the Taguchi method and grey relational analysis for the multi-response optimisation problem to cope with the demand. The key performance indicators used to achieve the optimum response characteristics were the grey-relational grade and the Taguchi's orthogonal array. A comprehensive framework that utilises TOPSIS (Technique for Order of Preference by Similarity to Ideal Solution), fuzzy logic, and ANN, respectively, in ranking, quantifying uncertainties and predicting performance is proposed. In achieving optimal global results, differential evolution (DE), big-bang big-crunch (BB-BC) algorithm and harmony search algorithm (HAS) are introduced, fused with ANN in all cases, and the comparison of the hybrids is reported. We found that the differential evolution algorithm performed better than BB-BC and HSA. The principal novelty of the paper is the unique introduction of Taguchi's approach and grey-relational analysis in performance analysis. In the current perspective, the applications of TOPSIS, fuzzy logic, and ANN are also novel. A third novel contribution is the introduction of optimisers in the model framework. It is concluded that this intelligent maintenance performance approach is applicable in industrial environments. The conclusion is supported by the results obtained from real-life manufacturing companies operating in Nigeria, utilised to validate the approach.
References
M. Koivupalo, M. Sulasalmi, P. Rodrigo and S. Vayrymen, “Health and safety management in a changing organisation: Case study global steel company,” Safety Science, vol. 74, pp. 128-139, 2015.
S. Khrais, T. Al-Hawani and O. Al-Araidah, “A fuzzy logic application for selecting layered manufacturing techniques,” Expert Systems with Applications, vol. 38, pp. 10286-10291, 2011.
L. Afkham, F. Abdi and A.R. Komijan, “Evaluation of service quality by using fuzzy MCDM: A case study in Iranian health-care centres,” Management Science Letters, vol. 2, pp. 291-300, 2012.
A. Lazim and N. Wahab, “A fuzzy decision making approach in evaluating ferry service quality,” Management Research and Practice, vol. 2, no. 1, pp. 94-107, 2010.
R. Kodali, R.P. Mishra and G. Anand, “Justification of world-class maintenance systems using analytic hierarchy constant sum method,” Journal of Quality in Maintenance Engineering, vol. 15, no. 1, pp. 47-77, 2009.
B. Hagg, “The great profitability potential in Swedish industry,” Proceedings of the 16th International Congress of Condition Monitoring and Diagnostic Engineering Management, pp. 19-22, 2003.
P. Ottosson, P. Torkkeh and O.P. Shirvastav, “Productivity and profitability analysis of an electric motor production line,” Proceedings of the 16th International Congress of Condition Monitoring and Diagnostic Engineering Management, pp. 767-772, 2003.
K.E. McKone, G.S. Roger and O.C. Kristy, “Total productive maintenance: A contextual view,” Journal of Operations Management, vol. 17, no.2, pp.123- 144, 1999.
G. Chand and B. Shirvani, “Implementation of TPM in cellular manufacturing,” Journal of Material Processing Technology, vol. 103, pp. 149-54, 2000.
C. Hwang, and K. Yoon, “Multiple Attribute Decision Making: Methods and Applications,” New York: Springer-Verlag, 1981.
T.J. Ross, “Fuzzy Logic with Engineering Applications,” John Wiley and Sons Ltd, West Sussex, England, 2004.
G. Taguchi, “Introduction to Quality Engineering,” White plains, New York: Kraus International Publications, 1986.
H-W. Wu and Z-Y Wu, “Using Taguchi method on combustion performance of a diesel engine with diesel/biodiesel blend and port-inducting H2,” Applied Energy, vol. 104, pp. 362-370, 2013.
M.Y. Wang and T.S. Lan, “Parametric optimisation on multi-objective precision turning using grey relational analysis,” Information Technology Journal, vol. 7, pp.1072-1076, 2008.
K.M. Subbaya, B. Suresha, N. Rayendra and Y.S. Varadarajan, “Grey-based Taguchi approach for wear assessment of SiC filled carbon-epoxy composite,” Materials and Design, vol. 41, pp. 124-130, 2012.
N. Natarajan and R.M. Arunachalam, “Optimisation of micro-EDM with multiple performance characteristics using Taguchi method and grey relational analysis,” Journal of Science and Industrial Research, vol. 70, pp. 500-505, 2011.
C-L. Yang, “Optimising the glass fiber cutting process using the Taguchi methods and grey relational analysis,” New Journal of Glass-Ceramics, vol. 1, pp. 13-19, 2011.
J. Moran, E. Granada, J. Miguez and Poterio, “Use of grey relational analysis to assess and optimise small biomass boilers,” Fuel Processing Technology, vol. 87, no. 2, pp. 123- 127, 2006.
Y.F. Hasio, Y.S. Tarang and W.J. Huang, “Optimisation of plasma arc welding parameters by using the Taguchi method with grey relational analysis,” Materials and Manufacturing Process, vol. 23, pp. 51-58, 2008.
K. Yiyo, Y. Taho and G-W. Huang, “The use of grey-based Taguchi method for optimising multi-response optimisation problems,” Engineering optimisation, vol. 4, no. 6, pp. 517-528, 2008.
C-P. Fung, “Manufacturing process optimisation for wear property of fibre-reinforced polybutylene terephthalate composites with grey relational analysis,” Wear, vol. 254, pp. 298-306, 2003.
E. Sari and A.M. Shaharoun, “A proposed maintenance performance measures for manufacturing companies,” Proceedings of the Asia Pacific Industrial Engineering and Management Systems Conference 2012 V. V. Kachitvichyanukul, H.T. Luong, and R. Pitakaso Eds, 2012.
A. Parida, T. Ageren and U. Kumar, “Integrating maintenance performance with corporate balanced scorecard,” Proceedings of the 16th International Congress of Condition Monitoring and Diagnostic Engineering Management, pp. 53-60, 2003.
P. Muchiri, L. Pintelon, L. Gelders and H. Martin, “Development of maintenance function performance measurement framework and indicators,” International Journal of Production Economics, vol. 131, pp. 295 – 302, 2011.
T. Sibalija, V. Majstorovic and M. Sokovic, “Taguchi-based and intelligent optimisation of a multi-response process using historical data,” Journal of Mechanical Engineering, vol. 57, no.4, pp. 357-365, 2011.
A. Parida, G. Chattopadhyay and U. Kumar, “Multi-criteria maintenance performance measurement: a conceptual model,” Proceedings of the 18th International Congress COMADEM, 31st Aug-2nd Sep 2005, Cranfield, UK, pp. 349-356, 2005.
A. Parida, “Study and analysis of maintenance performance indicators (MPIs) for LKAB. A case study,” Journal of Quality in Maintenance Engineering, vol. 13, no. 4, pp. 325-337, 2007.
R. Fritzsche, , J.N.D. Gupta & R. Lasch, “Optimal prognostic distance to minimise total maintenance cost: The case of the airline industry,” International Journal of Production Economics, vol. 151, pp. 76-88, 2014.
G. Waeyenbergh & L. Pintelon, “Maintenance concept development: a case study,” International Journal of Production Economics, vol. 89, no. 3, pp. 395-405, 2004.
Baluch, N. H., Abdullah, C. S., & Mohtar, S., “Maintenance management performance–An overview towards evaluating Malaysian palm oil mill,” The Asian Journal of Technology Management, vol. 3, no. 1, pp. 1-5, 2010.
R. Smith, “Key performance indicators: Leading or lagging and when to use them,” Available at www.reliabilityweb.com, assessed January 5, 2015.
A. Parida & U. Kumar, “Maintenance productivity and performance measurement,” In Handbook of Maintenance Management and Engineering (pp. 17-41). Springer, London, 2009.
D.E. Ighravwe & S.A. Oke, “A combined fuzzy goal programming and Big-Bang Big-Crunch algorithm for workforce optimisation with facility layout consideration,” Engineering Journal, vol. 19, no. 2, pp. 71-98, 2015.
D.E. Ighravwe & S.A. Oke, “A non-zero integer non-linear programming model for maintenance workforce sizing,” International Journal of Production Economics, vol. 150, pp. 204-214, 2014.
H.K. Alfares, “Aircraft maintenance workforce scheduling: A case study,” Journal of Quality in Maintenance Engineering, vol. 5, no. 2, pp. 78-88, 1999.
A. de Marco and G. Mangano, “A review of the role of maintenance and facility management in logistics,” Proceedings of the World Congress on Engineering 2012, vol. 3 WCE 2012, July 4 - 6, 2012, London, U.K., pp. 1-6, 2012.
A.H.C. Tsang, A.K.S. Jardine and H. Kolodny, “Measuring maintenance performance: a holistic approach,” International Journal of Operations and Production Management, vol. 19, no. 7, pp. 691-715, 1999.
G. Chen and T.T. Pham, “Introduction to Fuzzy System,” Chapman and Hall/CRC, London, 2006.
H.C. Lu and M-F. Yeh, “Robot path planning based on modified grey analysis,” Cybernetic System, Vol. 33, No. 2, pp 129-159, 2002.
H. Hasani, S.A. Tabatabaei and G. Amiri, “Grey relational analysis to determine the optimum process parameters for open-end spinning yarns,” Journal of Engineered Fibers and Fabrics. vol. 7, No. 2, pp. 81-86, 2012.
Z.W. Geem, J.H. Kim and G.V. Loganathan, “A new heuristic optimisation algorithm: Harmony search. Simulation, vol. 76, No. 2, pp. 60-68, 2001.
O.M. Alia and R. Mandava, “The variants of the harmony search algorithm: An overview. Artificial Intelligence Review, pp. 1-20, 2011.
K.L. Hsieh, “Parameter optimization of a multi-response process for lead frame manufacturing by employing artificial neural networks. International Journal of Advanced Manufacturing Technology, vol. 28, pp. 584-591, 2006.
R. Storn and K. Price “Differential evolution: A simple and efficient heuristic for global optimisation over continuous spaces. Journal of Global Optimisation, vol. 11, pp. 341-359, 1997.
A.P. Engelbrecht, “Artificial intelligence: An Introduction,” John Wiley, England, 2007.
O.K. Erol and I. Eksin, “New optimisation method: Big-bang big-crunch,” Advances in Engineering Software, vol.37, pp. 106-111, 2006.
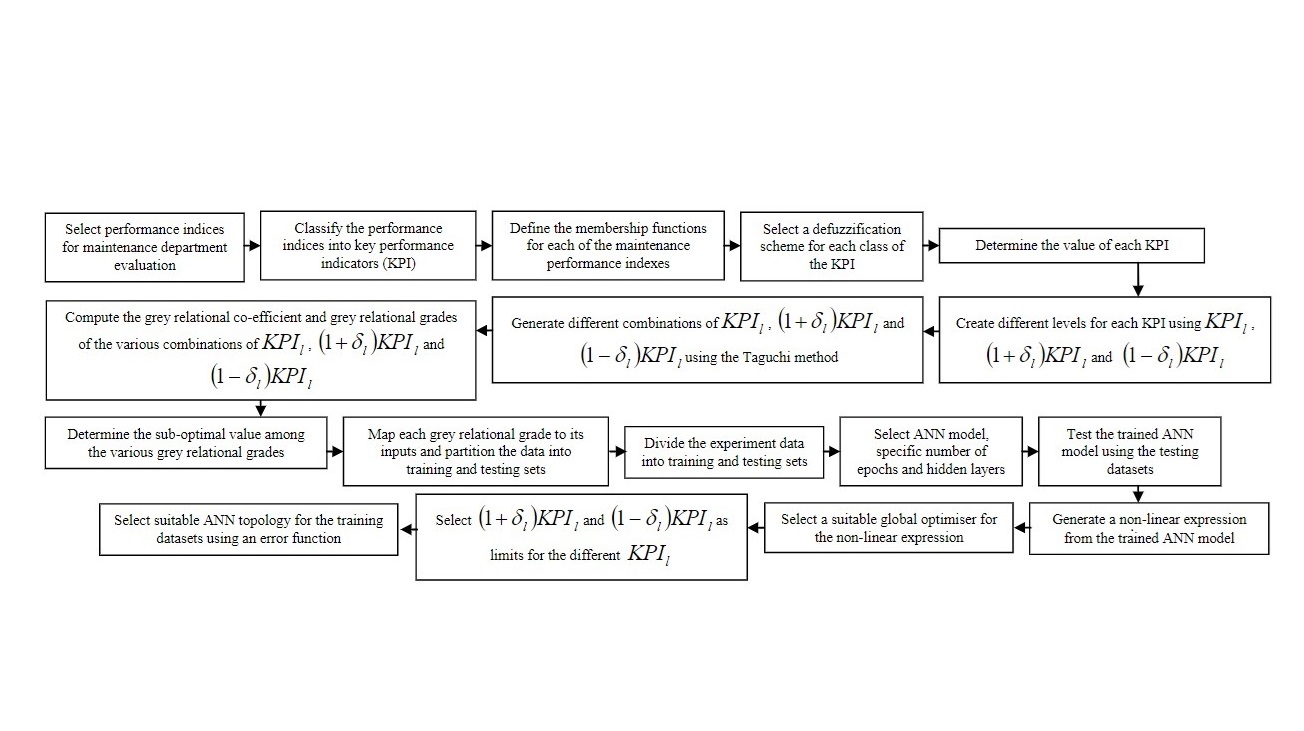
Downloads
Published
How to Cite
Issue
Section
License

This work is licensed under a Creative Commons Attribution-NonCommercial-NoDerivatives 4.0 International License.