Rice Yield Estimation Based on Machine Learning Approaches using MODIS 250 m Data
doi: 10.14456/mijet.2023.9
Keywords:
Machine learning, Rice yield, Modis 250 m, Remote sensingAbstract
Food security and water resource management depend on knowledge about the distribution of paddy rice fields. Information on rice production from space can be obtained using the technology of remote sensing. In the current study, the relationships between the rice spectrum, vegetation index, and rice yield can be assessed using the Moderate Resolution Imaging Spectroradiometer (MODIS). Machine learning has been evolving steadily in recent years, and its benefits are now readily apparent; particularly in the area of image processing, it is advancing quickly. The objective of this research is to estimate the rice yield using the MODIS satellite imagery data based on machine learning. Three machine-learning regression algorithms (multiple linear regression, support vector machine, and random forest) were evaluated, and a suitable model was created to estimate the rice production. According to the findings, the random forest model produced the most objective results, had the lowest RMSE values, and had good statistical correlations for both the training set and the test set. The methods described in this paper can be used as a reference for combining machine learning with MODIS satellite imagery data to estimate the rice yield in other locations.
References
P. Kulapramote, “Forecasting transplanted rice yield at the farm scale using moderate-resolution satellite imagery and the AquaCrop model: A case study of a rice seed production community in Thailand,”. ISPRS International Journal of Geo-Information, pp.72-73, 2018.
J. Jing and T. Guangjin. “Analysis of the impact of land use/land cover change on land surface temperature with remote sensing,” Procedia environmental sciences, pp.571-575, 2010.
R. Selçuk, “Analyzing land use/land cover changes using remote sensing and GIS in Rize, North-East Turkey,” Sensors, pp.6188-6202, 2008.
K. Abdulla-Al, et al. “Remote sensing approach to simulate the land use/land cover and seasonal land surface temperature change using machine learning algorithms in a fastest-growing megacity of Bangladesh,” Remote Sensing Applications: Society and Environment, vol.21, p.100463, 2021, doi:10.1016/j.envc.2021.100190.
Z. Qiqi, et al, “Land-use/land-cover change detection based on a Siamese global learning framework for high spatial resolution remote sensing imagery,” ISPRS Journal of Photogrammetry and Remote Sensing, pp. 63-78, 2022.
F. Steve, et al, “Agricultural land‐use in China: a comparison of area estimates from ground‐based census and satellite‐borne remote sensing: GCTE/LUCC RESEARCH LETTER,” Global Ecology and Biogeography, pp. 407-416, 1999.
H. Christoph, et al, “Best accuracy land use/land cover (LULC) classification to derive crop types using multitemporal, multisensor, and multi-polarization SAR satellite images,” Remote sensing, vol.8, pp. 684, 2016, doi:10.3390/rs8080684.
F. Steve, et al, “Combining remote sensing and ground census data to develop new maps of the distribution of rice agriculture in China,” Global Biogeochemical Cycles, pp. 38-1-38-10, 2002.
N. I. Wayan, N. Fumihiko and H. Chiharu, “Rice yield estimation using Landsat ETM+ data and field observation,” Journal of Agricultural Science, pp. 43-45, 2012.
S. A. Ali, D. Jan and B. Inbal, “Rice yield estimation using Landsat ETM+ Data,” Journal of Applied Remote Sensing, vol.9, pp. 095986-095986, 2015, doi:10.1117/1.JRS.9.095986.
A. M. Shew and G. Aniruddha, “Identifying dry-season rice-planting patterns in bangladesh using the landsat archive,” Remote Sensing, vol.11, p. 1235, 2019, doi:10.3390/rs11101235.
S. Terry and D. Tri and W. A. Wahyu, “Machine learning applied to sentinel-2 and landsat-8 multispectral and medium-resolution satellite imagery for the detection of rice production areas in Nganjuk, East Java, Indonesia,” International Journal of Remote Sensing and Earth Sciences, pp. 19-32, 2021.
F. Hongliang, et al, “Using NOAA AVHRR and Landsat TM to estimate rice area year-by-year,” International Journal of Remote Sensing, pp. 521-525, 1998.
N. I. Wayan, N. Fumihiko and H. Chiharu, “Relationship between rice spectral and rice yield using MODIS data,” Journal of Agricultural Science, vol.3, pp. 80-88, 2011, doi:10.5539/jas.v3n2p80.
S. Li, et al, “Monitoring paddy rice phenology using time series MODIS data over Jiangxi Province, China,” International Journal of Agricultural and Biological Engineering, pp. 28-36, 2014.
H. Jingfeng, “Remotely sensed rice yield prediction using multi-temporal NDVI data derived from NOAA's-AVHRR,” PloS one, vol.8, p. e70816, 2013, doi : 10.1371/journal.pone.0070816.
Z. Yuting, “Mapping paddy rice planting area in rice-wetland coexistent areas through analysis of Landsat 8 OLI and MODIS images,” International Journal of Applied Earth Observation and Geoinformation, pp. 1-12, 2016.
Z. Meng, “Mapping paddy rice using a convolutional neural network (CNN) with Landsat 8 datasets in the Dongting Lake Area, China,” Remote Sensing, vol.10, p.1840, 2018, doi:10.3390/rs10111840.
Q. Yuanwei, et al, “Mapping paddy rice planting area in cold temperate climate region through analysis of time series Landsat 8 (OLI), Landsat 7 (ETM+) and MODIS imagery,” ISPRS Journal of Photogrammetry and Remote Sensing, pp. 220-233, 2015.
H. Sotaro, “Maintaining higher leaf photosynthesis after heading stage could promote biomass accumulation in rice,” Scientific reports, vol.11, p. 7579, 2021, doi:10.1038/s41598-021-86983-9.
D. R. Panuju, “Mapping rice growth stages employing MODIS NDVI and ALOS AVNIR-2,” Remote Sensing and GIScience: Challenges and Future Directions, pp. 185-203, 2021.
I. M. Monirul, et al. Development of remote sensing-based yield prediction models at the maturity stage of boro rice using parametric and nonparametric approaches. Remote Sensing Applications: Society and Environment, vol.22, pp.100494, 2021, doi:10.1016/j.rsase.2021.100494.
Z. Lina, et al, “Machine learning on big data: Opportunities and challenges,” Neurocomputing, pp. 350-361, 2017.
D. C. A. Marcelo, et al, “The role of machine learning on Arabica coffee crop yield based on remote sensing and mineral nutrition monitoring,” Biosystems Engineering, pp. 81-104, 2022.
M. Gowhar, et al, “Assessing the yield of wheat using satellite remote sensing-based machine learning algorithms and simulation modelling,” Remote Sensing, vol.14, pp.3005, 2022, doi:10.3390/rs14133005.
K. R. THORP and D. E. N. A. Drajat, “Deep machine learning with Sentinel satellite data to map paddy rice production stages across West Java, Indonesia,” Remote Sensing of Environment, vol.265, p.112679, 2021, doi: https://doi.org/10.1016/j.rse.2021.112679.
M. Jong-Won, et al, “Regional-scale rice-yield estimation using stacked auto-encoder with climatic and MODIS data: A case study of South Korea,” International Journal of Remote Sensing, pp. 51-71, 2019.
S. Nguyen-Thanh, et al, “Machine learning approaches for rice crop yield predictions using time-series satellite data in Taiwan,” International Journal of Remote Sensing, pp. 7868-7888, 2020.
M. K. Mosleh, Q. K. Hassan, and E. H. Chowdhury, “Application of remote sensors in mapping rice area and forecasting its production: A review. Sensors (Switzerland),” pp.769– 791, 2015.
M. S. Mkhabela, P. Bullock, S. Raj, S. Wang, and Y. Yang, “Crop yield forecasting on the Canadian prairies using MODIS NDVI data. Agric. For. Meteo,” pp. 385-393, 2011.
N. T. Son, C. F. Chen, C. R. Chen, L. Y. Chang, H. N. Duc and L. D. Nguyen, “Prediction of rice crop yield using MODIS EVI-LAI -data in the Mekong Delta,” Vietnam. Int. J. Remote Sens, pp.7275-7292, 2013.
M. K. Mosleh, Q. K. Hassan, “Development of a remote sensing-based “Boro” rice mapping system,” Remote Sens., pp. 1938-1953, 2014.
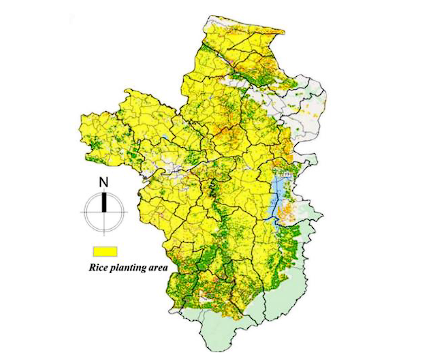
Downloads
Published
How to Cite
Issue
Section
License

This work is licensed under a Creative Commons Attribution-NonCommercial-NoDerivatives 4.0 International License.