Accuracy Enhancement of Consumer-Grade Global Positioning System (GPS) for Photogrammetric and City Mapping Determinations
Keywords:
city planning, UAV data, GPS accuracy, mapping location, KalmanAbstract
This paper address the fluctuation of a low-cost global GPS problem using an open platform of autonomous multi-rotors and particularly applying in location spotting from high accuracy UAV survey data. A city or urban planning requires a large scale of high precision survey data after data gathering using the photogrammetry method to regenerate the orthomosaic map. There was a problem when referring data from the map for access in real locations guided by a normal grade GPS receiver. The problem shows that the normal GPS cannot effectively work with high accuracy data. The aim of this study was to create a map location device using a normal GPS receiver for using high accuracy data from the industrial-grade UAV survey platform. We tested various GPS sensors applied with the Kalman filtering approach compared with the other source of field data then tuned the filter algorithm to improve the performance. The result shows that the Kalman filtering algorithm is presented significant reform to overcome the GPS data fluctuation problem and show a certain direction to perform the next step of a cost-effective map data pointer device.
Downloads
References
Beck, H., Lesueur, J., Charland-Arcand, G., Akhrif, O., Gagne, S., Gagnon, F., & Couillard, D. (2016). Autonomous takeoff and landing of a quadcopter. 2016 International Conference on Unmanned Aircraft Systems (ICUAS). doi:10.1109/icuas.2016.7502614
Diggelen, V., Frank, & Enge. (2015, September 18). THE WORLD’S FIRST GPS MOOC & WORLDWIDE LAB USING SMARTPHONES.
Kleeman, L. (n.d.). Understanding and Applying Kalman Filtering. Retrieved from https://ecse.monash.edu/centres/irrc/LKPubs/Kalman.pdf.
Langley, R. B. (1995). NMEA 0183: A GPS receiver interface standard. GPS world, 6(7), 54-57.
Sasiadek, J. Z., & Wang, Q. (2003). Low cost automation using INS/GPS data fusion for accurate positioning. Robotica, 21(3), 255-260. doi:10.1017/s0263574702004757
Julier, S. J., & Uhlmann, J. K. (1997). New extension of the Kalman filter to nonlinear systems. Signal Processing, Sensor Fusion, and Target Recognition VI. doi:10.1117/12.280797
Schmitz, G., Alves, T., Henriques, R., Freitas, E., & El’youssef, E. (2016). A simplified approach to motion estimation in a UAV using two filters. IFAC-PapersOnLine, 49(30), 325-330. doi:10.1016/j.ifacol.2016.11.156
Veness, C. (n.d.). Movable Type Scripts. Retrieved from https://www.movable-type.co.uk/scripts/latlong.html
Zhang, P., Gu, J., Milios, E., & Huynh, P. (n.d.). Navigation with IMU/GPS/digital compass with unscented Kalman filter. IEEE International Conference Mechatronics and Automation, 2005. doi:10.1109/icma.2005.1626777
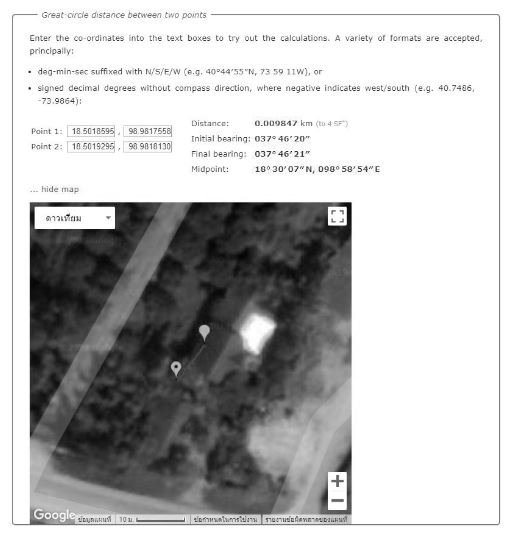
Downloads
Published
How to Cite
Issue
Section
License
Copyright (c) 2019 International Journal of Building, Urban, Interior and Landscape Technology (BUILT)

This work is licensed under a Creative Commons Attribution-NonCommercial-NoDerivatives 4.0 International License.